DaoAI Story
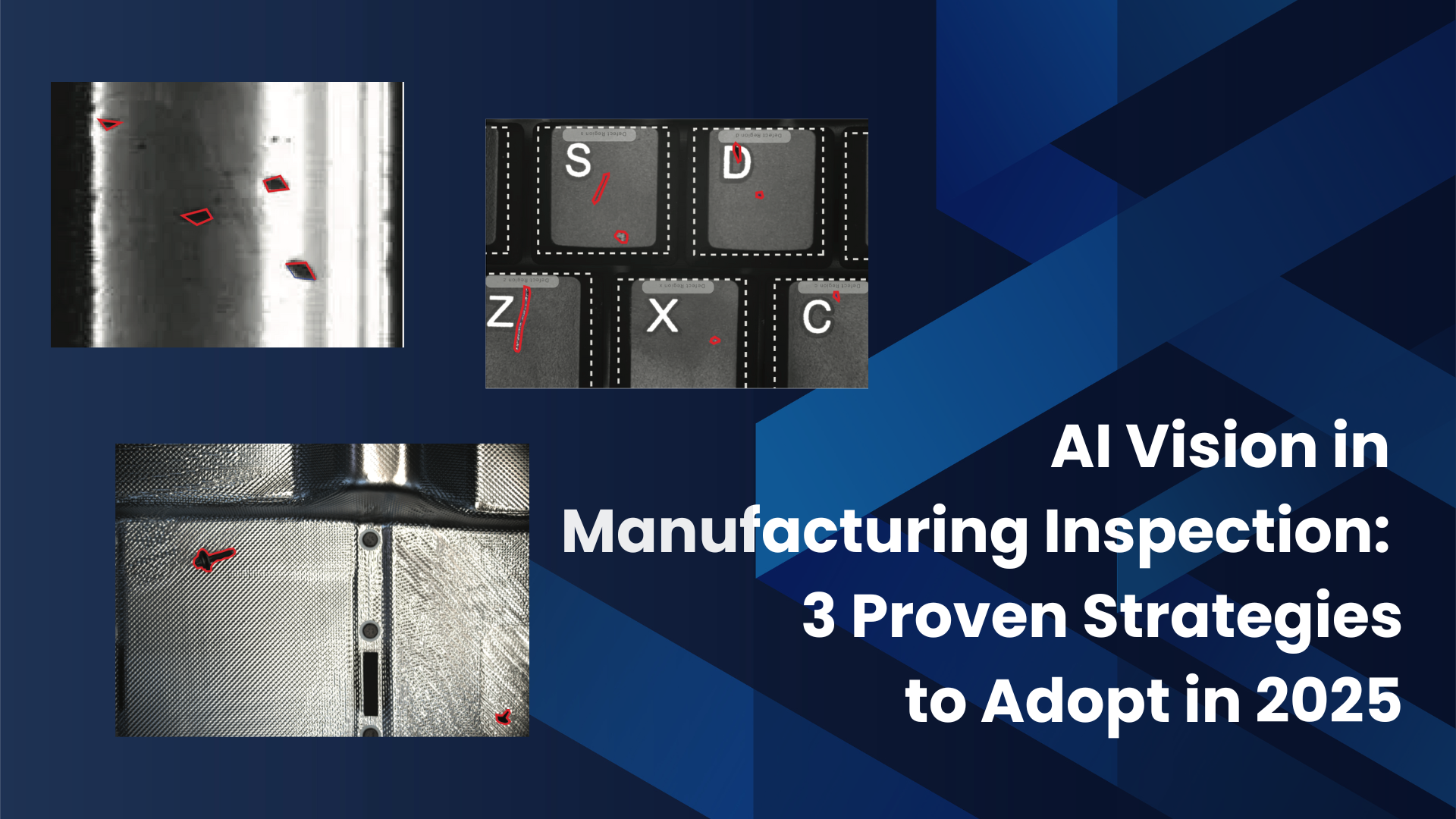
In the past year, manufacturers have eagerly embraced vision AI in their production lines—whether in industrial machinery, electronic product, or automotive.
The growing excitement around machine learning and AI has led to extensive experimentation across industries. However, while testing AI is relatively easy, the real challenge lies in scaling its impact and successfully integrating it into full production.
This is a transformative moment for AI in manufacturing, as past investments are now delivering significant results. One agricultural equipment manufacturer saved over $8 million per facility after implementing vision AI. Meanwhile, an AI-powered quality inspection system helped a manufacturer cut customer returns by half.
Effective Strategies for Implementing Visual AI in Manufacturing
- Assembly, packaging verification, Lining Verification
- Identifying quality issues and quality control
- Defect inspection and classification
Now, let’s explore these common challenges and look at real-world examples of manufacturers that have successfully overcome them with visual AI.
Ready to implement vision AI in your business? Contact with us
Solution 1: Avoiding Unknown Object in Assembly
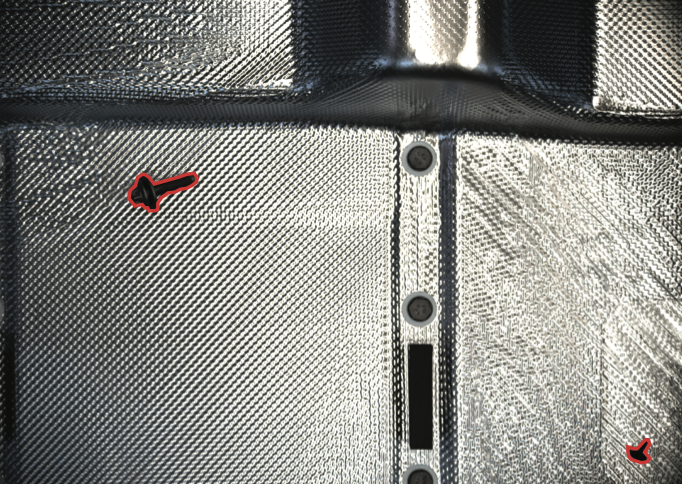
Our first story comes from a EV battery manufacturer. In assembly environments, residual or intrusive foreign objects are often unpredictable, making it even more challenging to collect all defect samples. By training an Unsupervised Defect Segmentation model using only positive samples, AI can automatically detect unidentified foreign objects, whether screws or hair, ensuring assembly quality.
Solution 2. Identifying Defects on Dark Surface Products
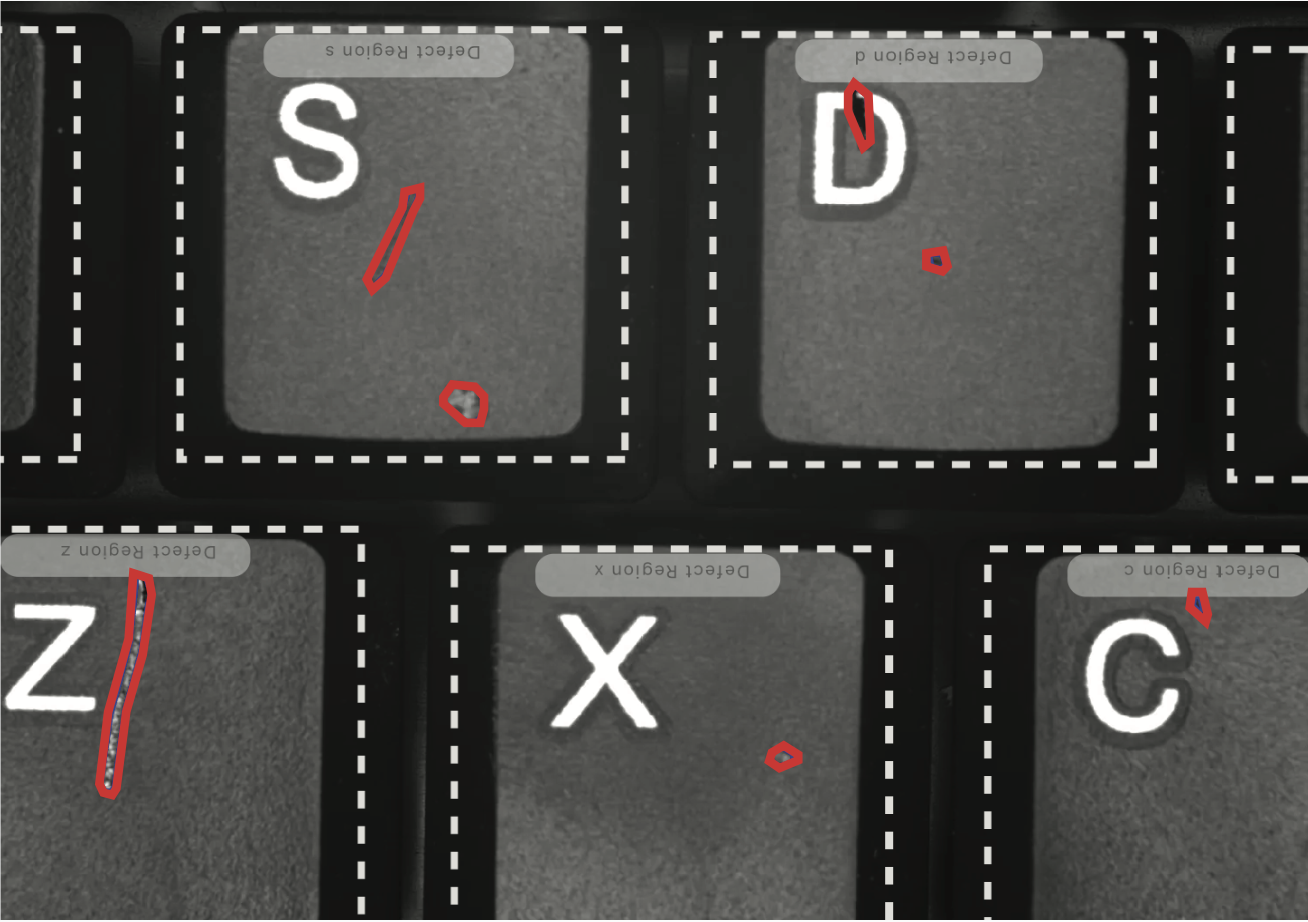
Our next story comes from a manufacturer of keyboard, common keyboards are typically black and may develop various defects on the keycap surface, such as faint scratches, chipped paint on printed text, or even fingerprints. By leveraging an Unsupervised Defect Segmentation model, we successfully trained the system to identify a total of 17 types of defects without defect annotation.
Solution 3. Identifying defects on high reflectivity surface
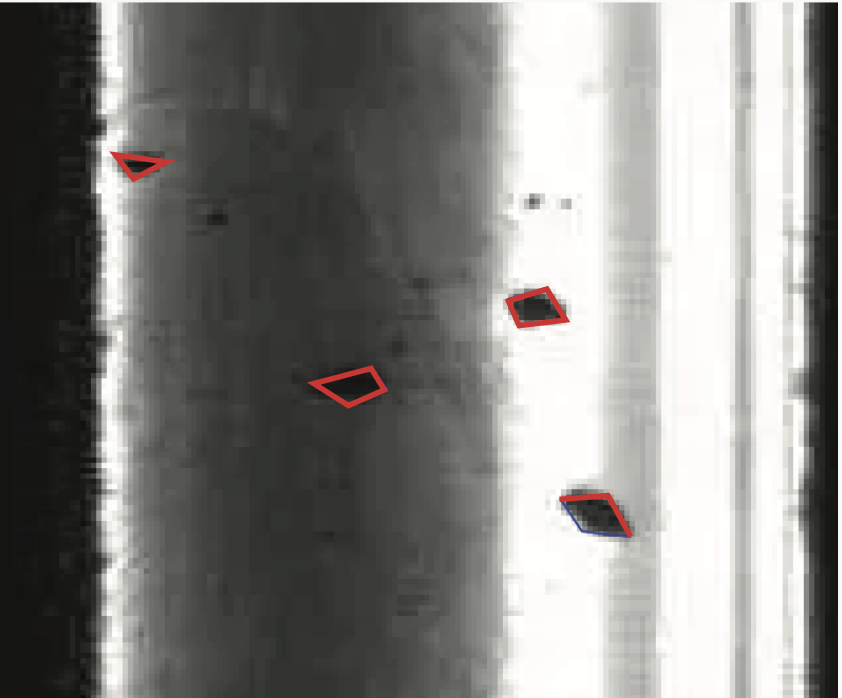
The high reflectivity of railway tracks and varying lighting conditions complicate training and judgment. DaoAI World enhances data with histogram equalization and manual image adjustments. The Unsupervised Defect Segmentation model, trained on defect-free samples, detects cracks, scratches, foreign objects, and corrosion. This unsupervised approach improves detection efficiency and accuracy without requiring extensive labeled data.
Driving Results with Vision AI – The Time is Now
From reducing unplanned downtime to improving product quality and strengthening workplace safety, these case studies highlight the real-world impact of visual AI in manufacturing.
As AI continues to evolve rapidly, manufacturers are poised to rethink their processes from the ground up. We look forward to seeing how this technology drives innovation and unlocks new solutions to complex business challenges in 2025.